A/B Testing: The Ultimate Guide to Conducting Impactful Experiments

A/B Testing: The Ultimate Guide to Conducting Impactful Experiments
A/B testing stands as a cornerstone in the realm of data-driven decision-making, allowing businesses and individuals to make informed choices about their digital strategies. By comparing two variations, A or B, of a single variable, testers can ascertain which one yields better performance in key metrics such as conversion rates or click-through rates. The method is grounded on the principle of isolating changes to ensure that results can be attributed directly to the variations being tested rather than external factors.

The process of A/B testing involves a series of meticulous steps, beginning with a hypothesis formation and followed by the execution of a controlled experiment. It is crucial in optimizing web pages, email campaigns, and various other aspects of a digital presence. The ultimate goal of A/B testing is to enhance user experience and increase the efficiency of conversion optimization. The insights gained from effectively designed and executed experiments serve as valuable inputs for continuous improvement in digital marketing and product development strategies.
Key Takeaways
- A/B testing allows for data-driven decisions by comparing variations of a single variable to improve key performance metrics.
- The testing process is methodical, from hypothesis to controlled experiment, ensuring results are attributable to tested changes.
- Effective A/B testing drives user experience enhancements and conversion optimization.
Understanding A/B Testing
A/B testing is a methodical approach that involves comparing two versions of a webpage or app to determine which one performs better. It's grounded in a hypothesis-driven process aimed at making data-informed decisions.
What Is A/B Testing?
A/B testing, also known as split testing, is a scientific method used to compare two versions of a single variable, typically by testing a subject's response to variant A against variant B, and determining which of the two variants is more effective. This approach allows for making careful changes to a user experience while collecting data on the results.
The Importance of Hypothesis in A/B Tests
In A/B testing, formulating a hypothesis is crucial as it gives direction to the experiment. A well-constructed hypothesis proposes a relationship between a specific variable change and a potential outcome. This prediction is testable and sets the stage for either validation or refutation through the testing process.
Variables and Variations
Key components of A/B tests include variables and variations. Variables are elements that can be changed or adjusted, such as headlines, images, or call-to-action buttons. Variations, on the other hand, are the actual changes made to these variables. Each variation is used to create a different version of what is being tested to see which performs better.
Control Groups and Statistical Significance
A control group is essential in A/B testing as it provides a baseline to compare against the variations. The control is the existing version (Version A), while the modified version is the challenge (Version B). Assessing the outcomes involves looking at statistical significance, which helps determine if the results are due to the changes made or simply due to random chance. Only when results reach a certain level of statistical significance can one conclude with confidence that the variations have had a real, measurable impact.
Setting Up Your A/B Test
Proper setup is crucial for conducting A/B tests that yield actionable insights. One begins by crafting experiments with precision, selecting relevant metrics for success, and calculating the necessary sample size to reach statistical significance.
Designing Effective Experiments
One designs an A/B test by creating two versions of a single variable to compare against each other – a control (the original) and a variant (the new version). Ensuring that the difference between the control and the variant is only in one key element prevents confounding results. This isolation makes it clear which element impacts user behavior.
Choosing Metrics and Establishing Goals
Determining the right metrics is foundational to measure the impact of an A/B test accurately. These could range from conversion rates to average time on page. Goals should be Specific, Measurable, Achievable, Relevant, and Time-bound (SMART). They provide clarity on what success looks like and help align the experiment with the business's overarching objectives.
Determining Traffic and Sample Size
An adequate sample size is necessary to detect a true difference between the control and variant. One must estimate the size based on the desired power of the test, the expected effect size, and the baseline conversion rate. Traffic allocation is equally important – deciding what percentage of the audience will see the control versus the variant. A balanced distribution is typically preferred to mitigate any bias in the experiment’s results.
Executing the Test
When conducting A/B testing, precision in execution is critical. It ensures accurate data collection and reliable results that can inform future decisions.
Segmenting Your Audience
Segmentation is crucial for targeting specific groups within the audience to determine how different variables affect behavior. Defining segments can be based on demographics, behavior, or even past interactions with the product. By segmenting the audience, one ensures that the differences in the campaign's performance are attributable to the variable being tested rather than external factors.
Running the Test
To properly run the A/B test, one must serve the control group (Group A) with the original version of the variable and the experimental group (Group B) with the new version. It's important to run both versions simultaneously to account for variables such as time of day or day of the week. This parallel testing helps in gathering data that is uncompromised by external time-related factors.
Monitoring and Adapting
Throughout the test, performance data must be continuously monitored. If anomalies or unexpected behaviors are detected, making a tweak in real-time may be necessary. However, one should be cautious not to make hasty adjustments that could compromise the integrity of the test. Patience is key; even if initial data suggests significant trends, wait until the test run is complete to draw conclusions.
Analyzing Test Results
Analyzing A/B test results is a critical stage where data tells a story. This process involves carefully examining the data to draw conclusions and make informed decisions.
Interpreting Data and Statistical Results
Analyzing an A/B test typically begins with an examination of the statistical results. One must determine if the outcome reflects a statistically significant difference between the variations tested. The significance is usually measured through p-values and confidence intervals, indicating the probability that the observed results are not due to chance. For example, if a variation in a website design leads to a higher conversion rate, one would check if the results are statistically significant before concluding that the new design is more effective.
Learning from the Data
Learning from A/B test results means looking beyond the surface to understand why one variation performed better than the other. It can be helpful to segment the data further, perhaps by user demographic or behavior, to gain insights into which factors influenced the outcome. The goal is to derive actionable insights that can guide future tests and optimize performance.
When to Trust the Results
Results can be deemed trustworthy when they are consistent and replicable. One should look for a sizeable sample that remains steady over a test period, reducing the impact of external factors and random variations. It's generally advised to run the test until the results reach statistical significance, at which point changes can be implemented with confidence. Furthermore, repeating the test or using validated data from similar experiments can reaffirm the results' reliability.
Advanced A/B Testing Techniques
In the realm of experimentation, advanced A/B testing techniques go beyond the basics to optimize and understand user experiences on a deeper level. These methods allow testers to experiment with multiple variables, assess the effect on complex user interactions, and prioritize what changes will have the most impactful results.
Multivariate and A/B/N Testing
Multivariate testing is a technique that extends upon traditional A/B testing by examining the impact of multiple variables simultaneously. This approach is useful for understanding how different elements interact with each other and affect user experience. A/B/N testing, a variation of A/B testing, involves comparing one control against multiple variations to identify which performs best. This method can offer deeper insights than standard A/B tests, especially when testing across a broader range of options.
Prioritizing Experiments
Prioritizing which experiments to run is critical in making efficient use of resources and time. Factors to consider include the potential impact on key metrics, the relevance to business objectives, and the feasibility of implementation. By employing a rigorous prioritization framework, teams can ensure that they focus on tests that are most likely to drive meaningful improvements in user behavior and experience.
Evaluating Complex User Interactions
Understanding complex user interactions and their impact on user behavior is essential for nuanced user experience optimization. Advanced A/B testing techniques allow practitioners to track and assess how users engage with different elements and which pathways lead to desired outcomes such as increased conversions or engagement. Evaluating these interactions often requires sophisticated analysis tools and a deep understanding of user psychology.
Improving User Experience and Conversion
Effective A/B testing is key to enhancing user experience, which in turn can lead to improved conversion rates. By systematically examining user interactions, it's possible to refine website elements to foster higher engagement and minimize obstacles that might prevent conversions.
Optimizing for Engagement
Engagement is a critical measure of a user's interest and interaction with a website. It's quantified through metrics like time on page, pages per visit, and event completions. To optimize for engagement, one should consider A/B testing different layouts, color schemes, and content positioning. For instance, testing horizontal vs vertical navigation bars can provide insights into user preferences and the navigational structures that best retain their attention.
Reducing Friction and Bounce Rate
Bounce rate is a metric that represents the percentage of visitors who leave a site after viewing only one page. High bounce rates can indicate friction in the user experience. A/B testing is useful for isolating and addressing the potential causes of friction. Elements to test could include form length, page load speeds, and clarity of calls-to-action. Adjustments in these areas can help create a more seamless user experience, engaging users long enough to consider a conversion.
Conversion Rate Optimization Best Practices
For conversion rate optimization (CRO), adhering to best practices is vital. These practices involve forming a hypothesis based on insights from user data, selecting an appropriate sample size, and ensuring statistical significance of the results. Testing elements like call-to-action button color and placement can reveal design choices that resonate with users. Utilizing A/B testing tools to analyze results helps identify which variations most effectively turn users into customers.
Operationalizing A/B Testing
Operationalizing A/B Testing involves establishing structured practices that intertwine the experimentation process with the core business workflows. It requires a comprehensive strategy where tools and platforms are leveraged to make data-driven decisions that could eventually reflect positively on product performance and business metrics.
Building an Experimentation Culture
It is essential for organizations to cultivate an environment where experimentation is viewed as a critical component of decision-making. This begins by educating team members about the value of A/B testing and its impact on business metrics. It extends to how a product can be enhanced through rigorous testing. Teams should be encouraged to test hypotheses and learn from each other’s experiments, creating a knowledge-sharing ecosystem that values continuous improvement.
Integrating A/B Testing into Business Workflows
A/B testing should be seamlessly integrated into the existing workflows to avoid disrupting business operations. This integration ensures that experiments are not isolated incidents but a part of the routine that contributes to refining the product. For instance, before any new feature is rolled out, it could be subject to A/B testing, with results analyzed using tools like Google Analytics to determine its effectiveness based on actual user data.
Role of Tools and Platforms
The choice and application of tools and platforms are pivotal in the experimentation process. They provide the functionality needed to design, implement, and analyze tests effectively. When choosing a platform, one should look for features such as easy integration with the product's technology stack, the ability to track a variety of business metrics, and robust reporting capabilities that translate data into actionable insights.
Next Steps after Testing
Once the A/B testing is complete and the results are analyzed, it is essential to leverage the insights gained to enhance product features and make strategic decisions. The data collected should inform the development of new features, and guide the broadening of the optimization program.
Applying Learnings to New Features
To ensure the continuous improvement of a product, one must apply the learnings from A/B tests to the development of new features. If the test revealed that users engage more with a simplified interface, future features should echo this preference. The key is to use data to support the design and user experience decisions, thereby reducing the risk of subjective bias.
Scaling and Expanding the Optimization Program
Scaling an optimization program involves systematically applying successful strategies across different areas of the product or to other products within the portfolio. Here’s how this can be approached:
- Identify winning elements from the A/B test
- Implement these elements in similar contexts or features
- Test at a larger scale to validate that improvements hold across different user segments
- Use the tested elements as a benchmark for future A/B tests
Through scaling, one can maximize the impact of successful optimizations, ensuring that enhancements benefit a wider audience and contribute to the overall success of the product.
Frequently Asked Questions
Understanding the intricacies of A/B testing can empower you to optimize your website or product effectively. The following frequently asked questions aim to clarify common uncertainties surrounding the process.
What are the essential steps for conducting effective A/B testing?
For effective A/B testing, one must first clearly define the objective and select a variable to test. Creating control (A) and variant (B) groups is crucial, with the goal of ensuring that these groups are as similar as possible, barring the variable under examination. Deploy the test and monitor the performance continuously to gather sufficient data. An understanding of when to conclude the test is equally important, which usually depends on achieving statistical significance.
How can statistical methods be applied to analyze online A/B testing results?
Statistical methods are pivotal in analyzing A/B testing results, as they provide a framework to infer whether observed differences are due to chance or actual effects of the variant. Techniques such as hypothesis testing, calculation of p-values, and confidence intervals are often used to determine the significance of the results. These methods help ascertain if the variant significantly outperforms the control, guiding data-driven decision-making.
How can A/B testing be integrated into the data science workflow?
Integration of A/B testing into the data science workflow begins with problem formulation, followed by the design of a controlled experiment. Data scientists must ensure proper randomization and adequate sample size to yield reliable results. After conducting the experiment, the analysis phase involves applying statistical models to interpret the data. If results are conclusive, they can inform the iterative process of model refinement and decision-making, thereby closing the loop in the data science lifecycle.
Stay up to date with our blog
Dive into our informative and engaging blog posts to stay informed on the latest trends in the Webflow & Shopify world as well on actionable tips to make your website work for you.

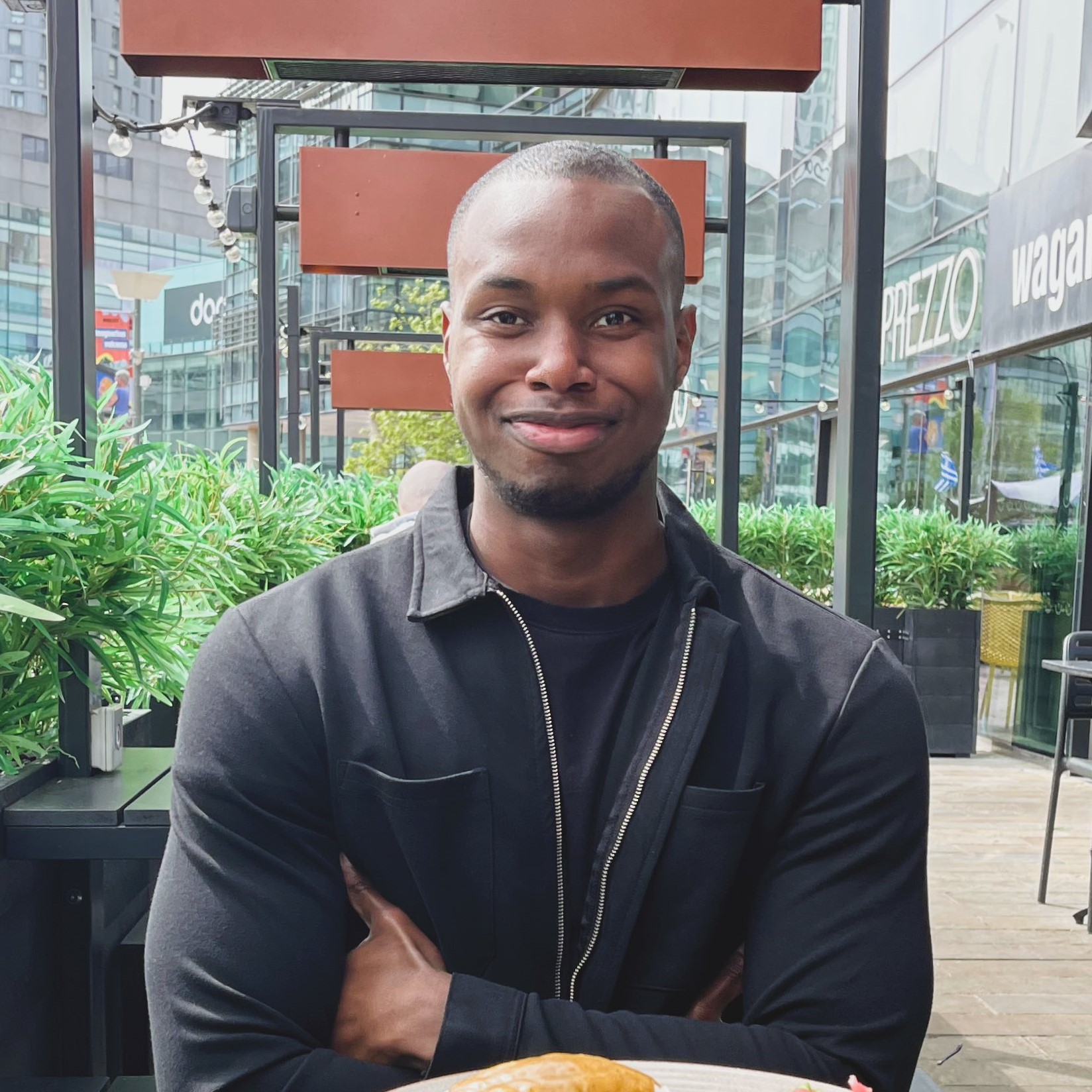
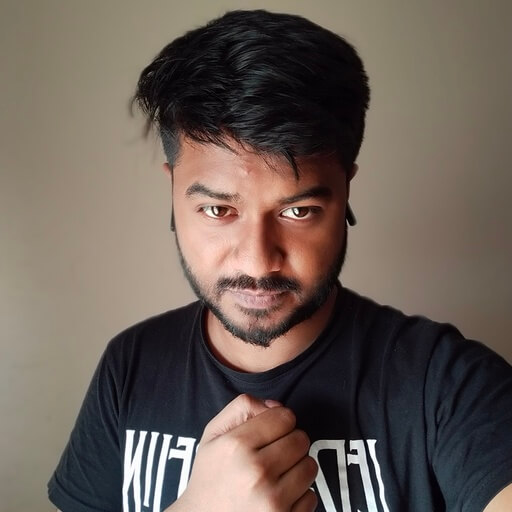
Contact us
We're only 1 email, call, message or meeting away. We'd be happy to help with your query. Book in a time on our calendar so we can speak.